Transforming Business Efficiency with a Machine Learning Labeling Tool
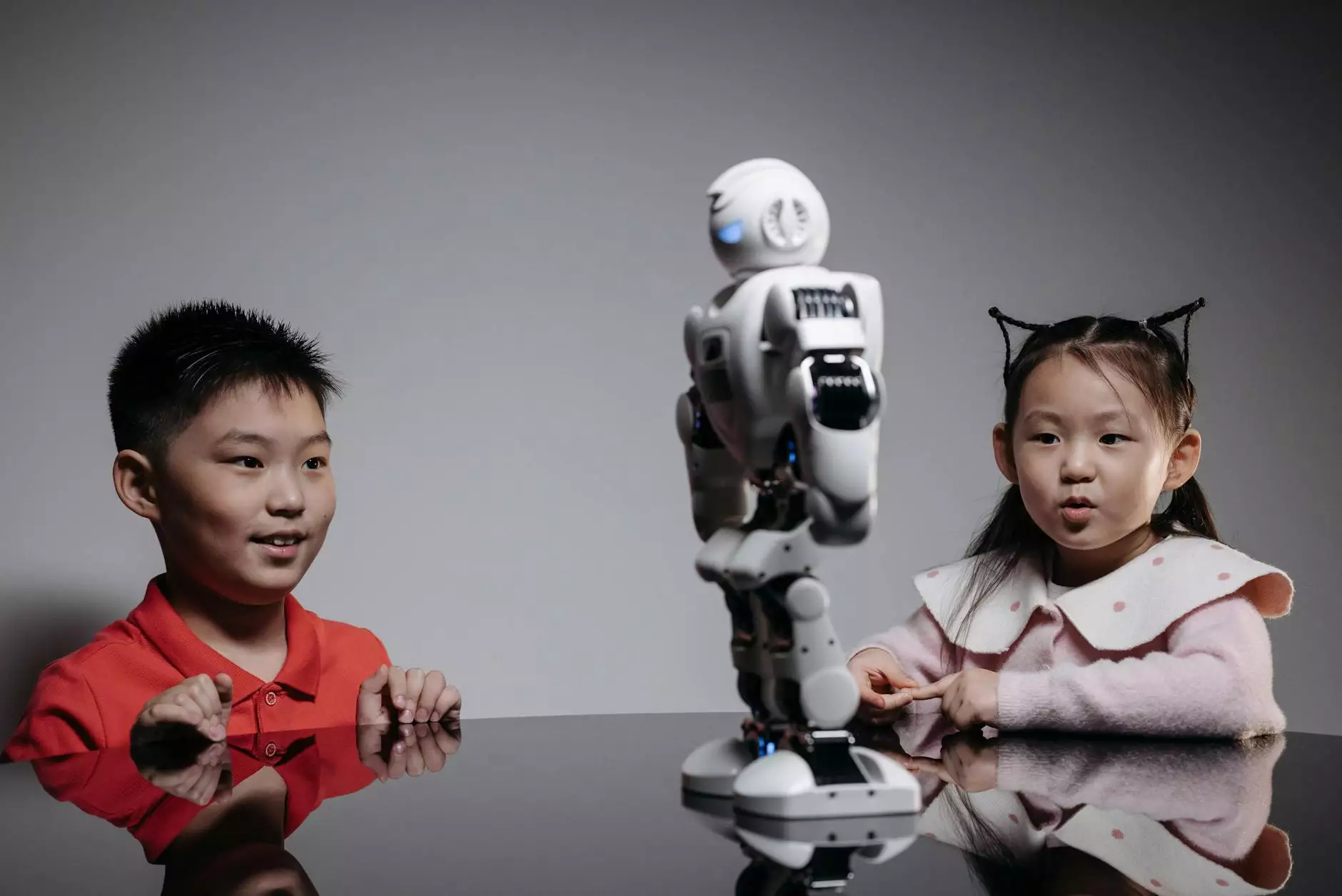
In the dynamic world of technology, machine learning is rapidly emerging as a game-changer for businesses across various sectors. Central to the effectiveness of machine learning algorithms is the quality and precision of the data on which they are trained. This is where a machine learning labeling tool comes into play. It not only streamlines the data annotation process but also improves the overall quality of machine learning models. In this article, we will delve deep into the significance of data annotation, the benefits of using a machine learning labeling tool, and how it can elevate your business strategies.
Understanding the Importance of Data Annotation
Data annotation is the process of labeling data to make it suitable for machine learning algorithms. It's an essential step, as it provides context to the data points that the algorithms utilize to learn and make predictions. There are several types of data that require annotation, including:
- Image Data: Identifying objects, faces, or other relevant features by drawing bounding boxes or segmentation masks.
- Text Data: Categorizing texts, tagging parts of speech, or annotating sentiments.
- Audio Data: Transcribing voice inputs or labeling sound effects.
- Video Data: Tagging actions, objects, or even emotions within video frames.
Proper annotation is crucial because the quality of the annotations directly impacts the performance of machine learning models. High-quality data leads to better insights and improved decision-making for businesses.
What is a Machine Learning Labeling Tool?
A machine learning labeling tool is a software solution designed to facilitate the efficient and accurate annotation of datasets. These tools automate much of the process, making it faster and easier for businesses to prepare data for machine learning purposes. Key features often include:
- Automated Annotation: Utilizing algorithms to pre-label data, which human annotators can then refine.
- Collaboration Tools: Enabling teams to work together seamlessly, regardless of location.
- Quality Control Measures: Ensuring annotations are accurate through various validation techniques.
- Integration Capabilities: Connecting with other software tools and platforms for streamlined workflows.
By implementing a machine learning labeling tool, businesses can overcome many challenges associated with traditional annotation methods, such as high costs and time consumption.
Benefits of Using a Machine Learning Labeling Tool
Adopting a machine learning labeling tool offers a multitude of benefits that can significantly enhance the way data is handled and utilized in business settings. Here are some key advantages:
1. Enhanced Efficiency
Manual data annotation can be a tedious and labor-intensive process. A labeling tool automates much of this work, reducing the time required to annotate datasets significantly. This acceleration enables companies to launch products faster and respond more swiftly to market changes.
2. Improved Accuracy
With built-in validation and quality assurance features, a machine learning labeling tool minimizes human errors, ensuring that the annotated data is reliable and trustworthy. Better accuracy in data leads to superior model performance and business outcomes.
3. Cost Effectiveness
Although there might be an initial investment required for implementing a machine learning labeling tool, the long-term savings are substantial. Reducing the amount of time and human resources needed for data annotation translates directly to cost savings for the business.
4. Scalability
As your business grows, so does the volume of data. Machine learning labeling tools can scale according to your needs, allowing you to handle larger datasets without compromising on the quality of annotations.
5. Versatility
Machine learning labeling tools are versatile and can be used for various types of data, making them suitable for different industries, including finance, healthcare, retail, and technology.
How to Choose the Right Machine Learning Labeling Tool
With numerous machine learning labeling tools available on the market, selecting the right one can be a challenge. Here are key factors to consider:
1. User-Friendly Interface
The tool should be intuitive and easy to navigate, allowing your team to start annotating data without a steep learning curve.
2. Feature Set
Evaluate the features offered by the tool, such as automated workflows, collaboration options, export formats, and integration capabilities with other tools you may already be using.
3. Customization options
Look for tools that allow you to customize the annotation process according to your specific needs, ensuring that it aligns well with your business objectives.
4. Support and Documentation
Reliable support and comprehensive documentation are crucial for troubleshooting and training purposes.
5. Pricing Structure
Make sure to understand the pricing model of the tool, whether it's subscription-based, pay-as-you-go, or a one-time purchase, and ensure it fits within your budget.
Real-World Applications of Machine Learning Labeling Tools
The impact of machine learning labeling tools spans various industries and applications:
1. Autonomous Vehicles
In the automotive industry, machine learning labeling tools are used for annotating images and videos collected from vehicle cameras to help cars understand and navigate their environments safely.
2. Healthcare
Machine learning in healthcare relies heavily on annotated medical images. Tools are employed to label x-rays, MRIs, and other medical test results, aiding in diagnostics and patient care decisions.
3. E-Commerce
In e-commerce, data annotation tools can help analyze customer sentiment through reviews and feedback, enabling businesses to adjust their offerings based on customer insights.
4. Surveillance and Security
Machine learning labeling is crucial in surveillance systems, where real-time analysis oflive footage helps in identifying threats or unusual behavior through annotated video feeds.
Conclusion
The incorporation of a machine learning labeling tool is no longer just an option but a necessity for businesses looking to thrive in the data-driven landscape. By enhancing efficiency, ensuring accuracy, and providing scalable solutions, these tools represent the future of data annotation. As organizations continue to recognize the transformative power of machine learning, investing in the right labeling tool, like those offered by Keylabs.ai, will be pivotal for achieving business excellence. Embrace this technology today and pave the way for a smarter, data-driven tomorrow.