AI Annotation Tools: Revolutionizing Software Development
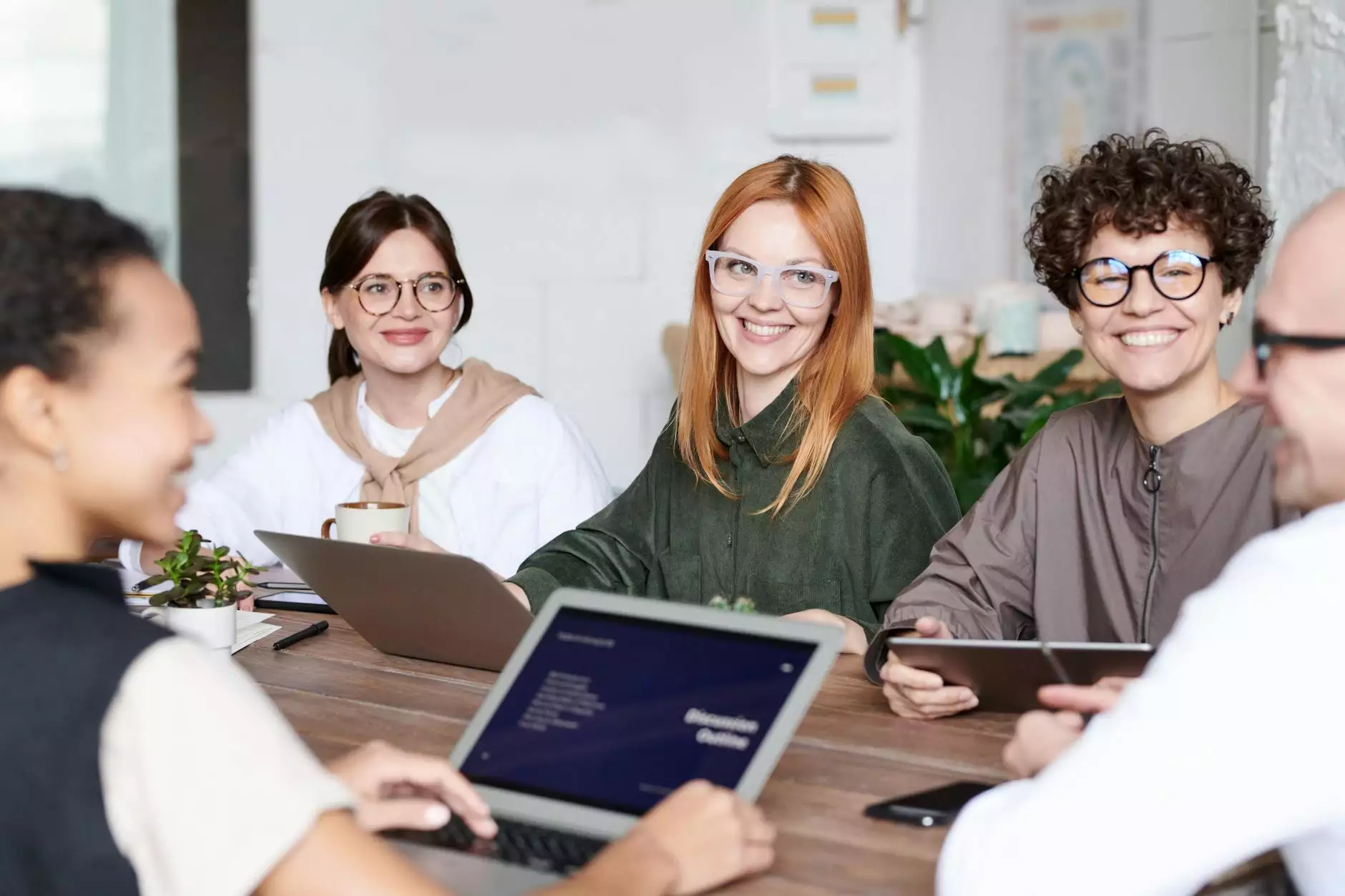
In the rapidly evolving landscape of technology, the integration of Artificial Intelligence (AI) into various facets of business operations has exponentially increased productivity and efficiency. One of the most significant advancements in software development is the advent of AI annotation tools. These tools are designed to facilitate the labeling and categorization of data, thereby enabling machine learning algorithms to function optimally.
Understanding AI Annotation Tools
AI annotation tools serve as pivotal instruments in training AI models. They automate the process of data labeling, which is essential for AI systems to understand and interpret the data they process. Without annotated data, machine learning models cannot learn or make predictions effectively.
What is Data Annotation?
Data annotation is the process of identifying and labeling data sets in a way that makes them meaningful for machine learning applications. Here are some common types of data annotations:
- Image Annotation: Labeling images for computer vision tasks, such as object detection and image classification.
- Text Annotation: Providing context to text data, including sentiment analysis or named entity recognition.
- Audio Annotation: Labeling audio clips for speech recognition and sound event classification.
- Video Annotation: Annotating video frames for tasks like activity recognition and motion detection.
The Importance of AI Annotation Tools in Software Development
The role of AI annotation tools in software development cannot be overstated. They streamline the data preparation pipeline, which is often a bottleneck in AI projects. The ability to quickly and accurately annotate data allows software developers to focus on building robust models that drive innovation.
Key Benefits of Using AI Annotation Tools
1. Enhanced Efficiency
AI annotation tools significantly reduce the time required to annotate data. Manual labeling is tedious and can lead to errors. With automated tools, the annotation process is not only faster but also more reliable.
2. Cost-Effectiveness
While there is an initial investment in AI annotation tools, the long-term savings on labor costs and project timelines can be substantial. Automating the annotation process can lead to a rapid return on investment.
3. Improved Accuracy
Automated tools are less prone to human error, leading to a higher quality of annotated data. Better data quality translates into improved model performance.
4. Scalability
The volume of data that businesses need to analyze is growing exponentially. AI annotation tools enable organizations to scale their data annotation processes in line with their needs, allowing them to handle large datasets efficiently.
Applications of AI Annotation Tools
The applications of AI annotation tools span various industries. Below are some prime examples:
Healthcare
In the healthcare sector, AI annotation tools assist in annotating medical images for diagnostic purposes. For instance, tools can help radiologists quickly identify tumors in X-rays or MRIs, potentially leading to early diagnosis and better patient outcomes.
Autonomous Vehicles
The development of self-driving cars relies heavily on computer vision. AI annotation tools label the training data that enables vehicles to recognize road signs, pedestrians, and other vehicles, ensuring safer navigation.
Retail
In the retail industry, businesses use AI annotation tools to analyze customer behavior through video footage. This analysis aids in optimizing store layouts and enhancing customer experiences.
Choosing the Right AI Annotation Tool
When selecting an AI annotation tool, businesses should consider several factors:
- User Interface: The tool should have an intuitive UI that simplifies the annotation process.
- Supported Data Types: Ensure that the tool supports the specific types of data you need to annotate.
- Integrations: Check if the tool can seamlessly integrate with your existing software and data pipelines.
- Customizability: Some projects may require specific annotation types; choose a tool that allows for customization.
- Cost: Evaluate the pricing model and choose one that fits your budget without compromising quality.
The Future of AI Annotation Tools in Software Development
As the demand for AI solutions continues to rise, the importance of AI annotation tools will only increase. Future developments may include:
1. Machine Learning Enhancements
Next-generation annotation tools may leverage machine learning to improve their own accuracy and efficiency. Self-learning algorithms could potentially learn from previously annotated data, reducing the need for human intervention.
2. Enhanced Collaboration Features
With teams distributed across different locations, future tools may include enhanced collaboration features that allow multiple users to annotate data simultaneously, thereby accelerating the process further.
3. Greater Adaptability
AI annotation tools of the future will likely become even more adaptable to different industries and use cases, providing tailored solutions that meet specific business needs.
Conclusion
In conclusion, AI annotation tools are transforming the landscape of software development by making data annotation faster, cheaper, and more accurate. As we continue to embrace the power of AI, these tools will play a vital role in the evolution of technology across various sectors. Investing in the right AI annotation tool will not only enhance your development process but also ensure that your AI models are trained on high-quality, well-annotated data, leading to more successful outcomes.
For organizations looking to innovate and stay ahead in this competitive market, exploring AI annotation tools is essential. With the right tools in hand, the possibilities for what businesses can achieve with AI are limitless.